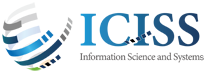
Prof. Fakhri Karray
IEEE Fellow
University of Waterloo, Canada & The Mohamed ben Zayed University of AI, UAE
Fakhri Karray is the inaugural co-director of the
University of Waterloo Artificial Intelligence
Institute and served as the Loblaws Research Chair
in Artificial Intelligence in the department of
electrical and computer engineering at the
University of Waterloo, Canada. He is also Professor
of Machine Learning and held the position of Provost
at the Mohamed bin Zayed University of Artificial
Intelligence (MBZUAI), in the UAE. Fakhri's research
focuses on operational and generative AI, cognitive
machines, natural human-machine interaction, and
autonomous and intelligent systems, with
applications to virtual care systems, cognitive and
self-aware devices, and predictive analytics in
supply chain management and intelligent
transportation systems. He aholds editorial roles in
major publications related to intelligent systems
and information fusion. Fakhri's latest textbook,
"Elements of Dimensionality Reduction and Manifold
Learning," was published by Springer Nature in early
2023. In 2021, he was honored by the IEEE Vehicular
Technology Society (VTS) with the IEEE VTS Best Land
Transportation Paper Award for his pioneering
research on enhancing traffic flow prediction using
deep learning and AI. Furthermore, his research on
federated learning in communication systems earned
him and his co-authors the 2022 IEEE Communication
Society's MeditCom Conference Best Paper Award.
Fakhri is also the co-founder and Chief Scientist of
Yourika.ai, a provider of AI-based online learning
systems. He holds fellowship status in the IEEE, the
Canadian Academy of Engineering, and the Engineering
Institute of Canada. Additionally, he has served as
a Distinguished Lecturer for the IEEE and is a
Fellow of the Kavli Frontiers of Science. Fakhri
earned his Ph.D. from the University of Illinois
Urbana-Champaign, USA.
Prof. Hong Zhu
Oxford Brookes University, UK
Dr. Hong Zhu is a professor of computer science at
the Oxford Brookes University, Oxford, UK, where he
chairs the Cloud Computing and Cybersecurity
Research Group. He obtained his BSc, MSc and PhD
degrees in Computer Science from Nanjing University,
China, in 1982, 1984 and 1987, respectively. He was
a faculty member of Nanjing University from 1987 to
1998. He joined Oxford Brookes University in
November 1998 as a senior lecturer in computing and
became a professor in Oct. 2004. His research
interests are in the area of software development
methodologies, including software engineering of
cloud-native applications, software engineering of
AI and machine learning applications, formal
methods, software design, software testing,
programming languages, software modelling, and
automated software engineering tools and
environments, etc. He has published 2 books and more
than 200 research papers in journals and
international conferences. He is a senior member of
IEEE, a member of British Computer Society and ACM.